Introduction When I was born, I was ignorant but very curious. I always asked
questions : "What? Where? Why? How?" And my parents guided me through
this jungle of knowledge, telling me about things that really mattered
and leaving what was complicated to the future.
As I grew up, I started learning about the very complex things by
cross referencing it with my already existent knowledge. If the new
incidents were not in my knowledge database, I queried the books,
articles and lately Google. Thus from an ignorant child with an empty
knowledge database, I grew up to become a knowledgeable person.
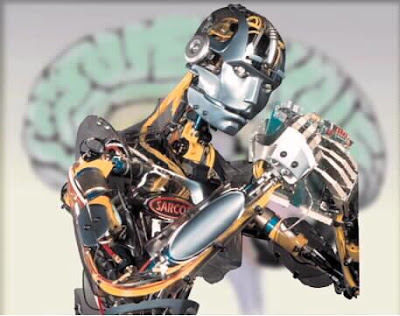
Let me take an example of how I learned English.
I am from India, English is neither my nor my parents' first language.
However, they really wanted me to learn how to read, write and speak
in English. They couldn't teach me by changing their mother tongue.
What they did, instead, was that they taught me the alphabets, a few
easy words and the basic grammar constructs. Then they gave me a
dictionary and a grammar book. I referenced new words that I saw with
the dictionary and new sentence formations with the grammar book. If I
still couldn't understand what was written, I asked my teacher.
Now when I come across anything written in English, I cross reference
it with the vocabulary and grammar that I have learned all these
years. If something new comes up, I check it up online or with my
peers. Thus I build upon my knowledge about English in an ongoing,
continuous process.
Implementation in Machine LearningNow, building on this very basic pattern how any human child learns
about this physical world, we can model this learning process for the
machines. This is a brief outline of the idea (here I take an example
of the English Language, but this is equally applicable to any other
natural language or any other real world entity):
"In the same way as I learnt about the world, Machines can also be
taught. In the same way that we teach a child how to read and
understand the English Language, a computer can be taught to recognize
patterns of English alphabets as words and can reference its meaning
with its dictionary (which is built by human assistance). The machine
can gradually build up its vocabulary by reading more and finding the
meanings of new words and sentence constructs online. If it finds
something which is complex enough, it can ask its human tutor its
meaning.
Given the amount of cheap storage, memory and computing power we have
in our hands today, I think it is a perfectly doable thing. I just
need time and resources to do it."
Value of this researchWhy is it important for a machine to learn a natural language? The
answer is simple, if machine learns a natural language, it can mine
the enormous amount of data on the internet to learn about any other
discipline. We will provide the world with the perfect student. It can
learn financial modelling from a human tutor and apply it to the
streaming financial data from the internet to predict future trends.
Maybe even produce its own models. It can learn biotechnology from its
human tutor and mine the huge available pathological data on the
internet to produce new medicines. The application possibilities seem
to be bound only by human imaginations.
Current Status of my ResearchRight now, I am working on a search engine which crawls the
blogosphere and indexes blogposts according to their emotional
quotient. Its a small step in the final scheme of things but this is
what I can do with the time and resources that I have (I have a '9 to
6' day job as a software engineer). I would love to devote more time
to this idea, given the mundane activities (like earning my bread and
butter) is taken care of :-).
I am not sure if this idea has been implemented previously anywhere.
Also, I acknowledge it is a very simple solution to a seemingly very
complex problem. However, I feel that this is the most suitable
solution. If we humans can do it then so can the machines.